How Machine Learning is Revolutionizing Business Analytics
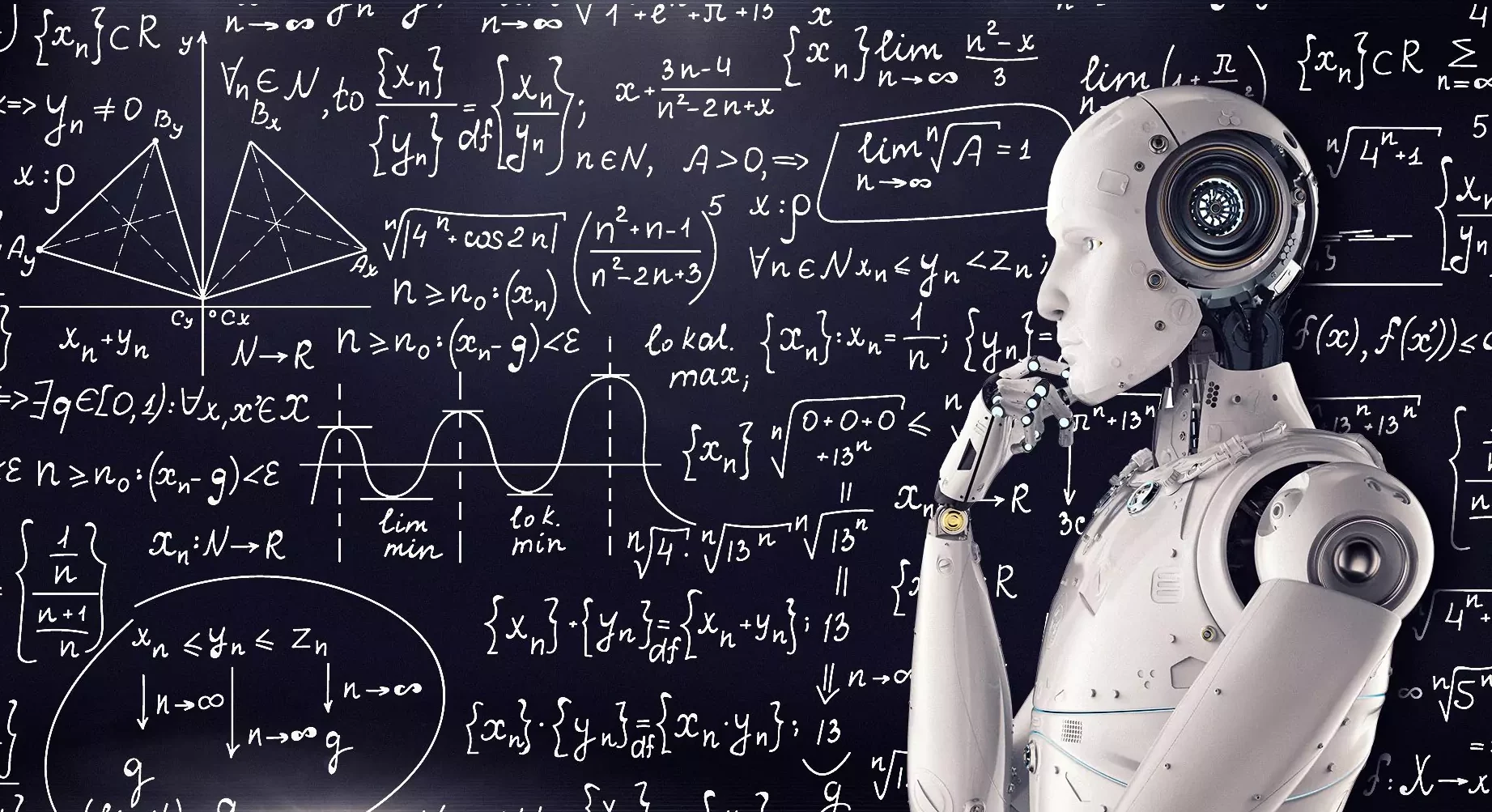
The business landscape is undergoing a profound transformation, driven by the integration of machine learning technologies into analytics processes. Organizations today are awash in data, but extracting meaningful insights that drive strategic decision-making has traditionally been complex and time-consuming. Machine learning is changing this paradigm, enabling businesses to uncover deeper insights, automate analytical processes, and predict future trends with unprecedented accuracy.
The Evolution of Business Analytics
Traditional business analytics relied heavily on historical data and backward-looking reporting. Analysts would identify patterns and trends manually, a process that was both labor-intensive and susceptible to human error. As businesses began collecting more data, these traditional methods became increasingly inadequate for delivering timely, actionable insights.
With the advent of machine learning, analytics has evolved from descriptive (what happened) and diagnostic (why it happened) to predictive (what will happen) and prescriptive (how we can make it happen). This evolution represents a fundamental shift in how organizations leverage data to drive business decisions.
Key Machine Learning Applications in Business Analytics
Machine learning has found numerous applications across the analytics spectrum, revolutionizing how businesses approach data-driven decision making:
- Predictive Analytics: ML algorithms can forecast future trends, customer behaviors, and market conditions based on historical data patterns, enabling proactive rather than reactive business strategies.
- Anomaly Detection: Advanced algorithms can identify unusual patterns that may indicate fraud, security breaches, or operational inefficiencies that might otherwise go unnoticed.
- Customer Segmentation: ML can discover nuanced customer segments by analyzing complex behavioral patterns, allowing for highly targeted marketing and personalization strategies.
- Sentiment Analysis: Natural Language Processing (NLP) algorithms can analyze customer feedback across various channels to gauge sentiment and identify emerging issues or opportunities.
- Demand Forecasting: ML models can predict future demand with greater accuracy by incorporating multiple variables like seasonality, economic indicators, and even weather patterns.
- Process Optimization: Reinforcement learning techniques help identify optimal operational processes, reducing costs and improving efficiency.
Transformative Benefits for Businesses
The integration of machine learning into business analytics offers several compelling advantages that are transforming how organizations operate and compete:
Enhanced Decision-Making
Perhaps the most significant impact of ML in business analytics is the improvement in decision-making quality. By processing vast amounts of data and identifying complex patterns, machine learning provides decision-makers with insights that would be impossible to discern through traditional analysis. These insights reduce uncertainty and allow for more confident decision-making.
Increased Operational Efficiency
ML automates many time-consuming analytical tasks, freeing up human analysts to focus on higher-value activities. This automation not only reduces costs but also increases the speed at which insights are generated and implemented, creating a more agile and responsive organization.
Personalized Customer Experiences
By analyzing customer data at a granular level, ML enables businesses to create highly personalized experiences. From product recommendations to customized marketing messages, this personalization increases customer satisfaction, loyalty, and ultimately, revenue.
Proactive Problem Solving
Traditional analytics identifies problems after they occur. ML-powered predictive analytics enables businesses to anticipate issues before they arise, allowing for preemptive action. This shift from reactive to proactive problem-solving can prevent revenue loss and maintain operational continuity.
Implementation Challenges and Best Practices
Despite its transformative potential, implementing machine learning in business analytics comes with several challenges:
- Data Quality and Preparation: ML models are only as good as the data they're trained on. Ensuring clean, comprehensive data is essential for accurate insights.
- Skill Gaps: Organizations often lack the specialized talent needed to develop and maintain sophisticated ML models.
- Interpretability: Complex ML models can function as "black boxes," making it difficult to explain how they reach specific conclusions.
- Integration with Existing Systems: Incorporating ML into established business processes and legacy systems presents technical challenges.
To overcome these challenges and maximize the value of ML in business analytics, organizations should consider these best practices:
- Start with clearly defined business problems rather than implementing ML for its own sake.
- Invest in data infrastructure to ensure high-quality, accessible data.
- Develop a data-driven culture that embraces analytical insights at all organizational levels.
- Consider explainable AI approaches when transparency in decision-making is critical.
- Build cross-functional teams that combine domain expertise with technical ML knowledge.
The Future of ML in Business Analytics
The integration of machine learning and business analytics is still in its early stages, with exciting developments on the horizon:
- Automated ML (AutoML): Tools that automate the end-to-end process of applying machine learning will democratize access to sophisticated analytics.
- Deep Learning Applications: More powerful neural network architectures will unlock insights from unstructured data like images, video, and natural language.
- Real-time Analytics: Edge computing and 5G will enable instantaneous analysis and decision-making based on streaming data.
- Augmented Analytics: ML will increasingly work alongside human analysts, automating routine tasks while enhancing human expertise for complex problems.
As these technologies mature, the line between human and machine analytics will blur, creating a new paradigm of augmented intelligence that combines the creativity and contextual understanding of humans with the pattern recognition and processing power of machines.
Conclusion
Machine learning is not just enhancing business analytics—it's fundamentally transforming how organizations extract value from data. By enabling more accurate predictions, deeper insights, and automated decision-making, ML is helping businesses operate more efficiently, serve customers more effectively, and gain sustainable competitive advantages.
Organizations that embrace this revolution, investing in the necessary infrastructure, talent, and cultural changes, will be well-positioned to thrive in an increasingly data-driven business landscape. Those that lag behind risk being outmaneuvered by more agile, data-savvy competitors who are leveraging ML to make better decisions faster.
The future of business analytics is not just about having data—it's about having the intelligence to transform that data into actionable insights. Machine learning is the key that unlocks this intelligence, making it an essential capability for forward-thinking organizations.